AlphaFold: Applications for Biomedical Research
AlphaFold is an AI tool developed by DeepMind, a subsidiary of Alphabet. Based on neural networks, it has the remarkable ability to predict the 3D structures of proteins from their amino acid sequences with near-experimental accuracy. The initial version of AlphaFold debuted in 2018 at the 13th Critical Assessment of Protein Structure Prediction (CASP13) competition, demonstrating the groundbreaking potential of deep learning in accurately predicting protein structures.
In 2020, AlphaFold2 was introduced at CASP14, far outperforming other tools in prediction accuracy. Jumper et al. primarily attribute this marked improvement to new network architectural features and training procedures “based on the evolutionary, physical and geometric constraints of protein structures.” In collaboration with the European Bioinformatics Institute (EMBL-EBI), DeepMind has made over 200 million of these structural predictions available to researchers through the AlphaFold DB.
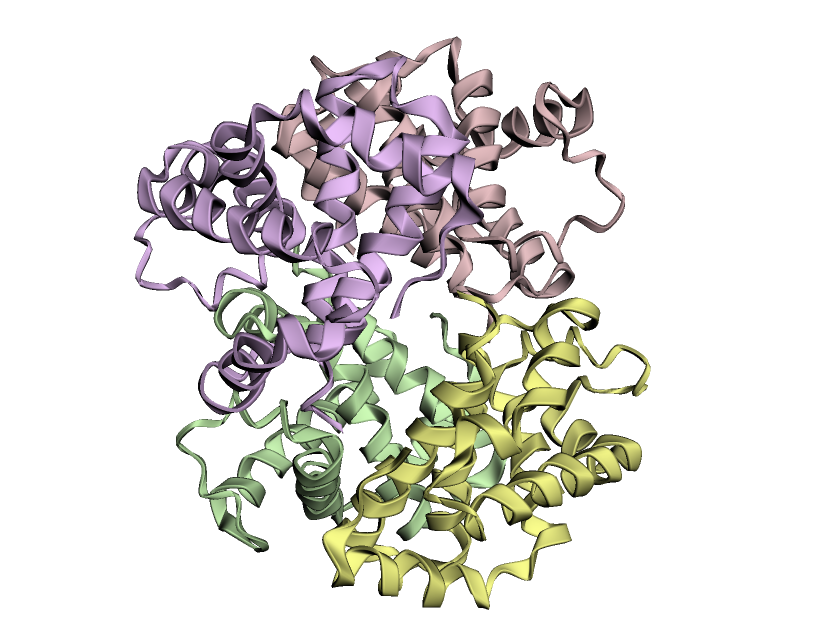
Why is AlphaFold seen as revolutionary in biomedical research?
Proteins and their binding sites are crucial targets for therapeutic intervention, and they can also be engineered to function as therapeutics themselves. A protein’s structure is key to comprehending its function in a biological system and its implications in health and disease. However, the amount of amino acid sequence data generated by high-throughput technologies has far outpaced scientists’ ability to determine how these amino acids are arranged in 3-dimensional space.
Without accurate computational tools, determining a single protein structure from its sequence may take years of experimental iterations using laborious and expensive techniques like X-ray crystallography and cryogenic electron microscopy (cryo-EM). Additionally, some proteins are not amenable to these experimental techniques (for example, some will not crystalize easily).
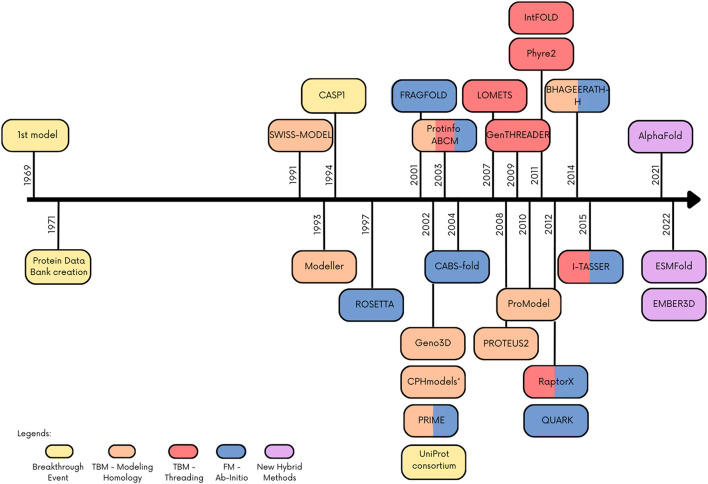
AlphaFold provides an invaluable tool for overcoming this bottleneck and accelerating drug discovery and development as well as fundamental research in structural biology. To demonstrate the potential of pairing protein structural data with large-scale sequencing datasets, the developers of AlphaFold also published a companion paper showing how the program can be used to accurately predict protein structures for the entire human proteome.
What are some key biomedical applications of AlphaFold?
First, AlphaFold offers a valuable tool to accelerate structure-based drug design. To effectively target a protein for therapeutic intervention, researchers need to understand its structure and design a molecule that will interact with it in the desired manner. For example, kinase inhibitors are commonly used to target protein kinases, which are known to be involved in the pathology of many diseases. Kinase activity is broadly regulated by switching between active and inactive conformations. Using AlphaFold to model the pharmacologically relevant structural features of kinases and their inhibitors, such as conformational diversity and docking performance, can improve the efficacy of these drugs.

The diversity of protein kinase conformations, compared between the RCSB PDB database (top) and AlphaFold database (bottom). From Al-Masri et al., “Investigating the conformational landscape of AlphaFold2-predicted protein kinase structures,” Bioinform Adv. (2023)
Second, AlphaFold predictions can help researchers streamline target identification for drug development. Some protein targets are more “druggable”, or able to be modified by therapeutic intervention, than others – and their 3D structure can be used to gauge druggability. For example, scientists studying the Hepatitis E virus (HEV) used AlphaFold to predict and explore 5 different structures for a replicase that is critical for the virus’ self-replication. Understanding features like the binding domains and enzyme boundaries of these structures, which have not yet been experimentally determined, is crucial for screening potential therapeutic targets.
Beyond the applications in small-molecule therapeutics discussed above, AlphaFold holds promise for advancing the development of “large-molecule” therapeutics, or biologics. Using larger molecules like antibodies as drugs poses unique challenges due to the complexity of understanding, modifying, and delivering these structures. However, antibody therapies also offer unique advantages, as they start with molecules that have naturally evolved to target specific proteins. AlphaFold can be a useful tool in exploring the relationship between antibody structure and potentially therapeutic properties.

Comparison of how protein therapeutics (i.e. biologics) and anti-CRISPR proteins act on their targets. From Park et al., “Rethinking Protein Drug Design with Highly Accurate Structure Prediction of Anti-CRISPR Proteins,” Pharmaceuticals (Basel) (2022).
Outside of the immediate realm of drug discovery and development, AlphaFold can also improve our understanding of the relationship between protein structure and disease. For example, an important feature of the SARS-CoV2 virus responsible for causing COVID-19 is its spike (S) protein, which helps the virus’s entry into host cells. This protein is the main target of neutralizing antibodies produced naturally by the body or introduced via antibody therapy, and exhibits mutations across different variants of the virus. By utilizing AlphaFold, researchers have modeled the structural variants of the S protein among various SARS-CoV-2 strains. These models provide valuable insights into the behavior of these variants within the body and their responsiveness to antibodies.
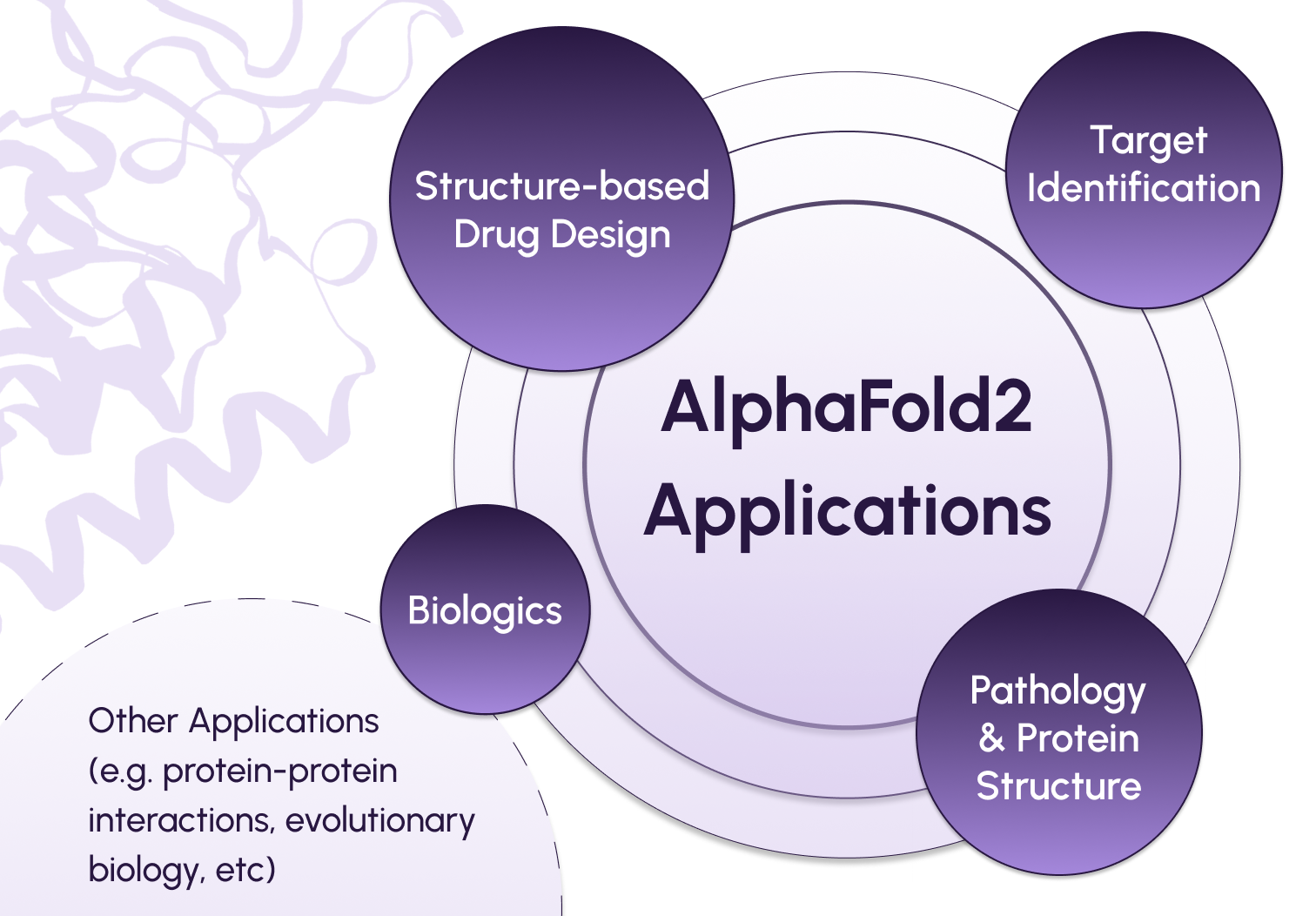
How can Watershed help you leverage AlphaFold?
While new machine learning tools promise to push the boundaries of biological inquiry, their practical applications often demand a considerable level of computational power and expertise. Watershed addresses these challenges by enhancing accessibility to AlphaFold2 and other ML-based tools in several key ways:
1. Our platform offers a user-friendly API for seamless interaction with AlphaFold2, incorporating features like caching and batch computing to optimize time and resource utilization.
2. We provide the compute power and infrastructure necessary both for processing large volumes of training data, and for running the prediction algorithm.
3. AlphaFold2 can be easily integrated with other workflows and pipelines in the Watershed platform.
4. Our team of bioinformatics experts is readily available to help you navigate AlphaFold2 as well as any data processing challenges that arise.
With Watershed, you can interface with AlphaFold2 with as much or as little support as you need. Plus, you can easily access human protein structures from AlphaFold DB without running the program at all.
Get in touch with our team at contact@watershed.bio to learn more.