Geneformer: Powering Drug Target Discovery with Network Biology
What is Geneformer?
Geneformer is a tool developed by Theodoris et al. (Nature 2023) to predict tissue-specific gene network dynamics from single-cell transcriptomic data. Pretrained on 30 million single-cell transcriptomes, Geneformer employs a specific kind of deep learning called transfer learning to glean contexts and relationships from this data, enabling it to make context-specific predictions in data-limited settings.

How can Geneformer and similar models be used for therapeutics research?
Building precision medicines requires a high-confidence understanding of the underlying molecular pathophysiology of a given disease. Drug hunters need to uncover how an illness perturbs signaling networks in healthy tissues before identifying and prioritizing targets for reversing a disease’s molecular phenotype.
Until recently, doing so required huge amounts of disease- and tissue-specific data. This can incur enormous time and capital costs for common disorders, or simply preclude using this approach for rare diseases or those with tissues inaccessible for study. Geneformer allows researchers to infer complicated gene interaction networks, without requiring existing data on the specific cell type or disease of interest.
In their paper, Theodoris et al. used Geneformer to identify candidate therapeutic targets for cardiomyopathy. The model predicted a set of genes whose activation or deletion would be likely to revert hearts with cardiomyopathy back to a healthy state. Remarkably, the team was able to experimentally validate several of the top hits from Geneformer’s in silico screen, demonstrating the far-reaching potential of using deep learning to transform public datasets into candidate therapeutic targets.
As an additional validation, the team was able to “synthetically reprogram” cells using Geneformer. By artificially adding a high-expression of OCT4, SOX2, KLF4, and MYC to a fibroblast gene signature, the authors observed Geneformer predicting a significant shift in other gene programs to an iPSC-like state, consistent with the state-of-the-art in producing iPSC in the lab.
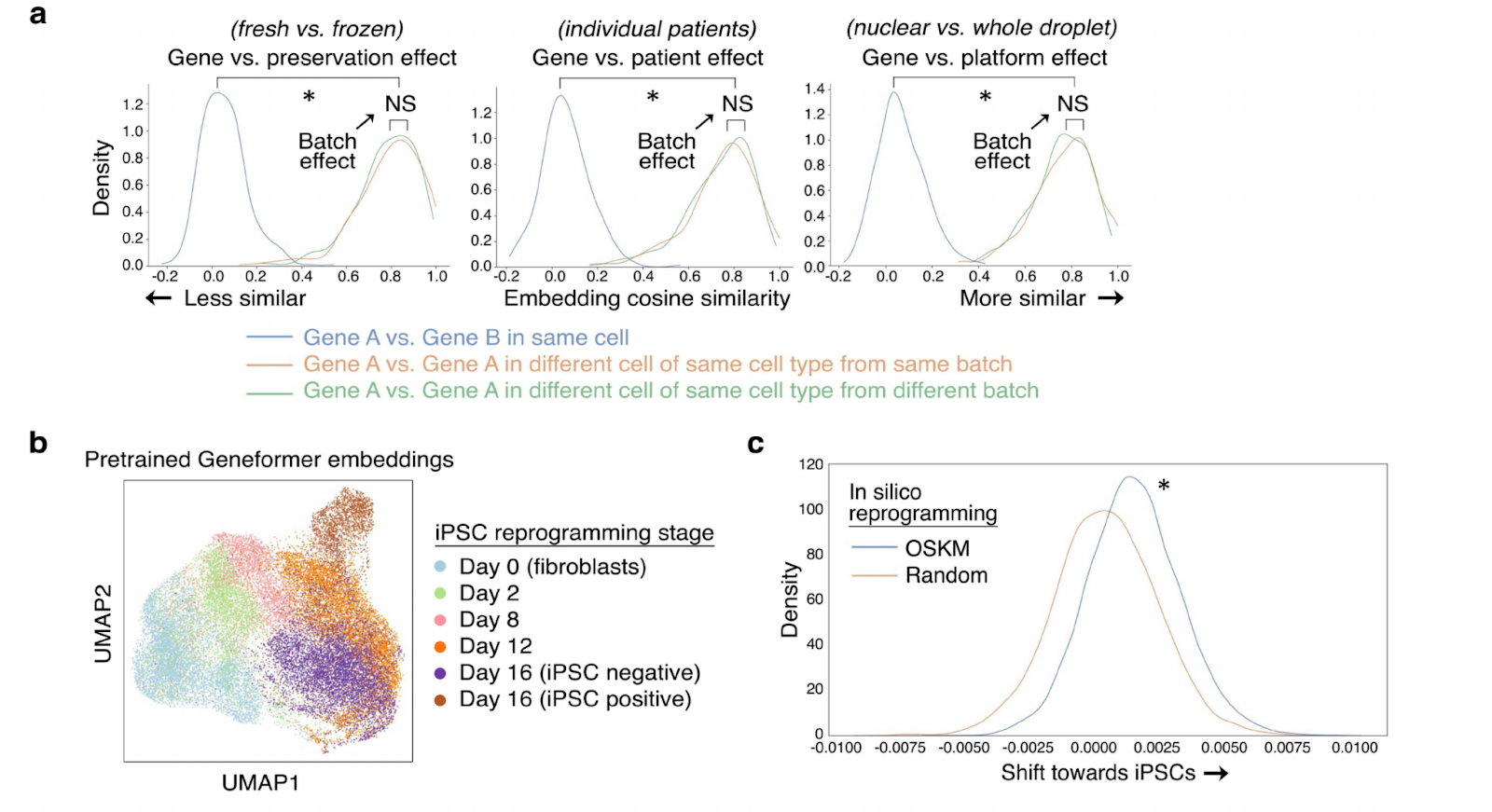
How does Geneformer enable predictions in data-limited settings?
A key characteristic of transformer models is their ability to learn general patterns from the data upon which they're trained and apply them to new datasets and questions. They take into account the specific context surrounding the input data, and understand the nuances around how over-expressing a given gene may yield different results in different cell types (i.e. cardiomyocytes versus astrocytes). Transformers can also be ‘fine-tuned’ to perform especially well on a task of interest, such as predicting therapeutic targets.
How can I leverage Geneformer in my own studies?
While Geneformer is publicly available, fully leveraging its potential – especially with fine-tuning tasks – requires interdisciplinary expertise across machine learning and data engineering, as well as access to powerful GPUs.
With Watershed you can fully harness the power of Geneformer because:
1. Watershed provides the compute power and infrastructure necessary for running the Geneformer algorithm to its fullest extent
2. Geneformer can be easily integrated with other workflows and pipelines in the Watershed platform.
3. Our team of bioinformatics experts is readily available to help you navigate the most appropriate uses of Geneformer for your applications, and solve any data processing challenges that arise.
If your team wants to use deep learning models like Geneformer to pursue your therapeutic hypotheses, email us at contact@watershed.bio to get in touch with our team of expert biologists, bioinformaticians, and machine learning engineers.