Leveraging Public Datasets for Drug Discovery
Drug discovery and development in 2025 won’t just be aided by public datasets and foundation models – it will be defined by them. This paradigm shift towards open datasets and data types has fueled an explosion of new large-scale research initiatives.

A few notable examples include:
- Open Targets: A platform combining population genetics with functional genomics screens in relevant cellular systems, which aims to enable systematic identification and prioritization of drug targets.
- JUMP-Cell Painting Consortium (JUMP-CP): An in-depth investigation of 116,750 unique compounds, 20,000 gene perturbation studies, and 1.6 billion cells in multiple disease systems. JUMP-CP allows for predictive targeting of pathways by specific compounds in order to determine drug action.
- AlphaFold2: A deep neural network for predicting the structure of a protein to atomic-scale resolution, using only its amino acid sequence. Trained on 100,000 3-dimensional protein structures, the network has predicted over 200 million proteins.
- GeneFormer: A transformer model for tissue-specific prediction of gene perturbation, trained on 30 million single-cell transcriptomes.
How can open data and models accelerate drug discovery?
Historically, understanding a disease well enough to develop a drug has taken decades. From drug discovery through regulatory approval, each step introduces its own unique bottlenecks.
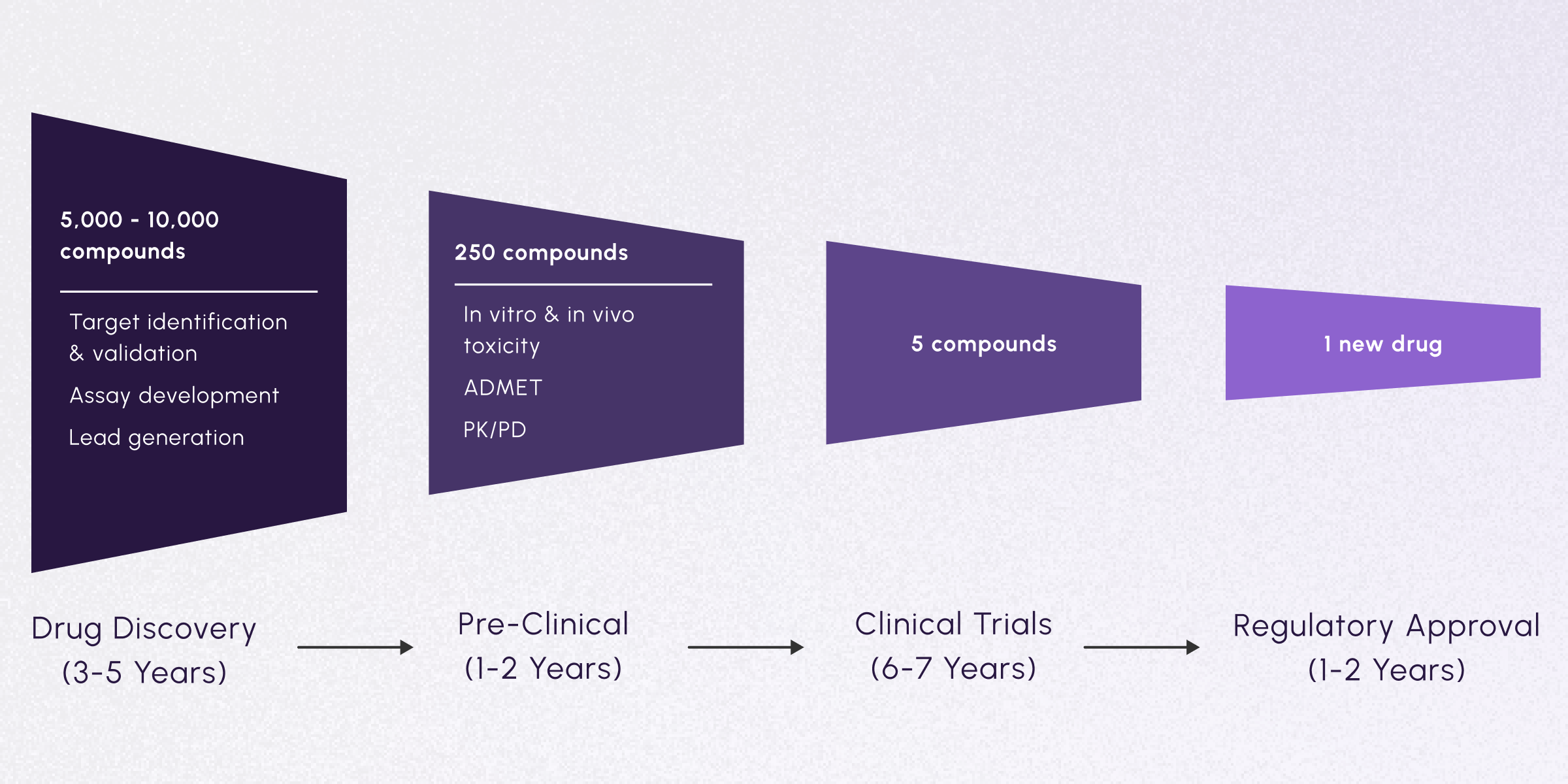
One of the most challenging steps in this process is the drug discovery phase: identifying and prioritizing therapeutic targets from thousands of candidate compounds. This consists of selecting which mRNAs, proteins, or pathways may be good targets for treating a disease. Typically, the data upon which these studies are based are incomplete, biased, and expensive as well as time-consuming to generate. Neglecting to integrate signals across different datasets and data types can further hinder insights and bias results. Open datasets hold the promise of allowing researchers to extract those signals in days instead of months.
What about the later stages of drug development?
After the drug discovery phase, only a few hundred compounds may move on to pre-clinical trials. In this step, compounds are tested in animal models to assess important characteristics like absorption, distribution, metabolism, excretion, and toxicity (through ADMET and PK/PD studies). From the remaining pool of candidates, less than 10 may be approved for clinical trials in human patients. Finally, if clinical trials show significant therapeutic potential with minimal adverse effects, 1 new drug may emerge for regulatory approval.
In both clinical and post-clinical studies, patient segmentation can help identify which patients are most likely to respond to a drug. There is a growing body of data available to help researchers delineate clinically salient patient subsets, including multi-omics data such as genomic, transcriptomic, and proteomic data. However, these must be effectively utilized in order to derive meaningful insights.
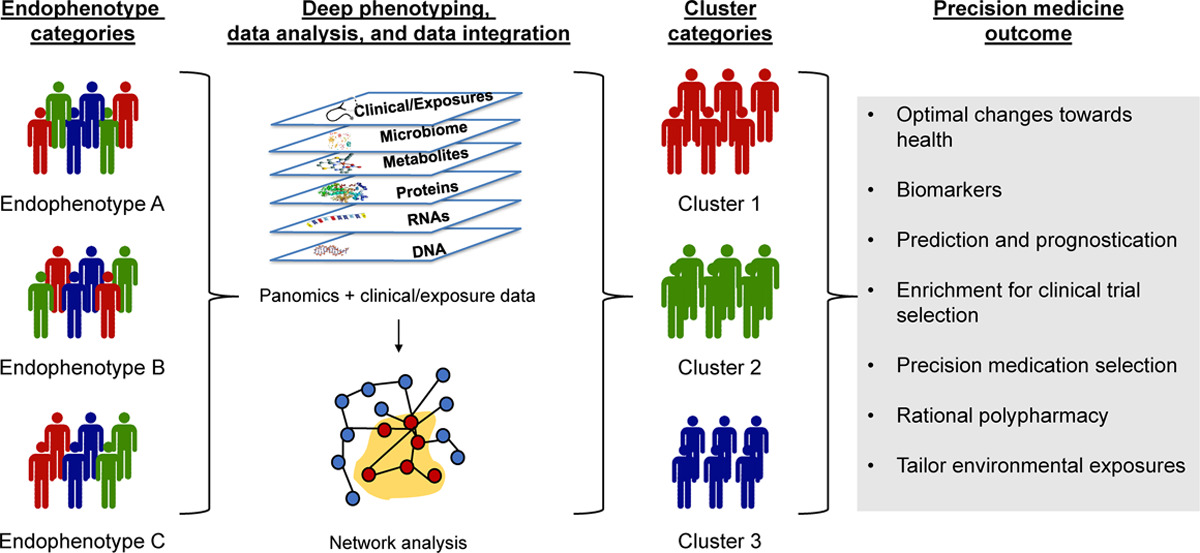
In a 2023 Cell study, Dr. Yize Li et al. demonstrated the power of integrating cohesive multi-omics data to make impactful, translatable discoveries in cancer research. The team used transcriptomic, proteomic, and phosphoproteomic data to identify clusters of oncogenic driver pathways across ten cancer types. Deeper analyses revealed important insights about therapeutic responses observed in the clinic.
If this is such a promising approach, why doesn’t everyone do it?
Effective integration and analysis of these diverse datasets requires a complex ecosystem of resources, including specialized infrastructure and multidisciplinary expertise.

Infrastructure needs to be secure and capable of handling protected patient data, as well as enable FAIR (findable, accessible, interoperable, reusable) data practices that are robust enough to withstand regulatory scrutiny. Mistakes in infrastructure can turn thousands of hours of effort into irreproducible noise. Even after appropriate infrastructure has been built, data need to be accurately manipulated and interpreted. PhD-level bioinformatics and data science expertise are absolute requirements for bespoke analyses, as entire drug programs can hinge on their results.
Finding and utilizing the right resources to effectively investigate data can be challenging, but it’s worth the effort to do it correctly! Watershed’s support and resources can powerfully advance your therapeutic program at every stage. We are prepared to grow with you from your first pilot dataset to productionisation of a therapeutic product and beyond. Our team is always available for input and guidance on data processing when you need it.